Breakthrough way to train neuromorphic chips
Using a biosensor to detect cystic fibrosis as the test case, TU/e researchers have devised an innovative way to train neuromorphic chips as presented in a new paper in Nature Electronics.
![[Translate to English:] [Translate to English:]](https://assets.w3.tue.nl/w/fileadmin/_processed_/9/7/csm_van%20Doremaele%20Banner%20image_8441cea27f.jpg)
Neuromorphic computers – which are based on the structure of the human brain – could revolutionize our future healthcare devices. However, their widespread use is hindered by the need to train neuromorphic computers using external training software, which can be time-consuming and energy inefficient. Researchers from Eindhoven University of Technology and Northwestern University in the US have developed a new neuromorphic biosensor capable of on-chip learning that doesn’t need external training. As a proof-of-concept, the researchers used the biosensor to diagnose cystic fibrosis based on sweat samples.
“We have demonstrated that we can create a ‘smart biosensor’ that could learn to detect a disease, such as cystic fibrosis, without using a computer or software.” That’s how Eveline van Doremaele summarized their new paper with Yoeri van de Burgt from TU/e, as well as Xudong Ji and Jonathan Rivnay from Northwestern University in the US that has just been published in Nature Electronics,
The ‘smart biosensor’ in their research is a neuromorphic biosensing computer – a device whose operation takes inspiration from the way that neurons communicate with other neurons in the human brain.
“Neuromorphic computing could have a significant impact on healthcare for example, particularly when it comes to point-of-care devices to check for an illness or condition,” says van Doremaele. “And in our research, we have solved a major problem with regards to the use of neuromorphic computers in healthcare.”
We have demonstrated that we can create a ‘smart biosensor’ that could learn to detect a disease, such as cystic fibrosis, without using a computer or software.
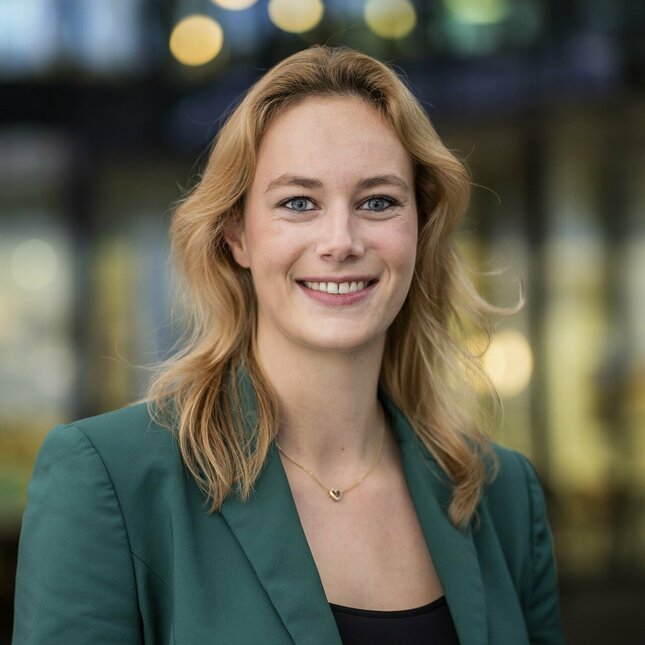
Goodbye to external software
So, what is the problem that van Doremaele and her collaborators solved? “For practical use in healthcare devices, neuromorphic technologies need to have low power requirements, interface with a sensor, and be easily trained for use. The first two of these can be solved with organic-based electronics. But it’s the training part that’s the central issue.”
Until now, a neuromorphic chip’s neural network would be trained using external software, which is a process that can be time-consuming and energy inefficient. “Now, our new chip can learn on-the-fly by processing patient data in real-time, which certainly speeds up the training process and helps promote the use of the chip in real interactive bioapplications,” says the researcher.
Searching for chloride anions
To test the effectiveness of their brand new chip, the researchers used it to test for the genetic disease cystic fibrosis. Cystic fibrosis is a hereditary disease that can damage organs, such as the lungs and digestive system.
One existing way to test for the disease is via a sweat test where a high level of chloride anions is an indicator of cystic fibrosis. Reliable sensors are already available to test for cystic fibrosis, so this test provided the researchers with an easy-to-check case study for their on-chip learning sensor.
“For ease of implementation, we didn’t work with real patient data. Instead, we used sweat samples from healthy donors,” says van Doremaele. “One sample was a negative sample or healthy sample of donor sweat, while a second sample was prepared to have a very high concentration of chloride anions.”
The researchers’ neuromorphic biosensor consists of three main parts – the sensor module, the hardware neural network, and the output classification part. A drop of sweat is added to the sensor module after which chloride and other ion concentrations in the sweat are detected with ion-selective electrodes. These signals are then processed by the neuromorphic chip itself. Finally, the result of the analysis is displayed as a green or red light indicating a negative or positive result, respectively.
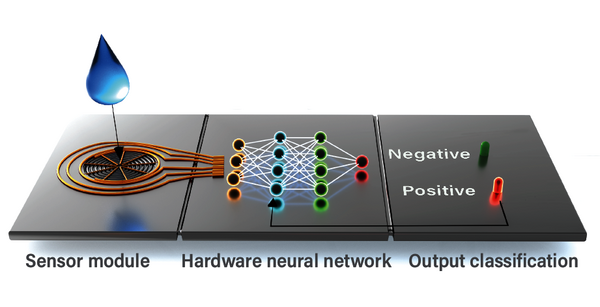
Training at the ‘data gym’
Before the chip was used to evaluate the main sweat samples, the neural network had to go the ‘data gym’ and undergo some supervised training.
“We created a number of sweat samples with varying and known ion concentrations and then tested the samples on the chip. If the result from the chip for a test was wrong, we corrected the chip, which resulted in corrections to the weights between the nodes of the neural network,” says van Doremaele. “Importantly, we train the chip on the hardware itself.”
This is the major advancement in this research – the ability to train the neural network on the chip and all without the need for any external software. “When the chip is trained to the problem of interest (here detection of cystic fibrosis from sweat samples), there is no further external control or intervention needed,” adds van Doremaele.
The ease of retraining
The real novelty is that the chips can learn and adapt to their application and environment.
In addition, even when trained, the chip can be used for another problem. “Say you want to use the same neural network hardware in a smart prosthetic hand or arm. All you have to do is retrain the neural network at the ‘data gym’ with information on hand or arm movements in this case,” says van Doremaele.
This new on-chip learning approach opens up the possibility of personalized implantable neural networks that are trained by the end user through the use of data directly from the user. “Such an approach to training neural networks for healthcare could have significant implications for people, and may someday provide a way to train chips in real-time to control prosthetics or other similar devices. The real novelty is that the chips can learn and adapt to their application and environment. They do not have to be programmed beforehand, as is the case today.”
Further information
A retrainable neuromorphic biosensor for on-chip learning and classification, van Doremaele et al., Nature Electronics, (2023).
Media contact
More on Health
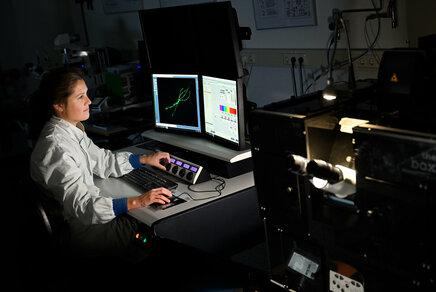
![[Translate to English:] [Translate to English:]](https://assets.w3.tue.nl/w/fileadmin/_processed_/e/9/csm_BvOF%20sluitstuk%20Luuk%20van%20Laake%203_c4df17db41.jpg)
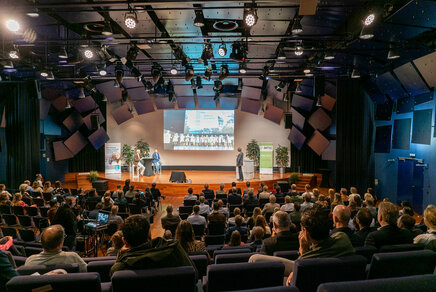
Latest news
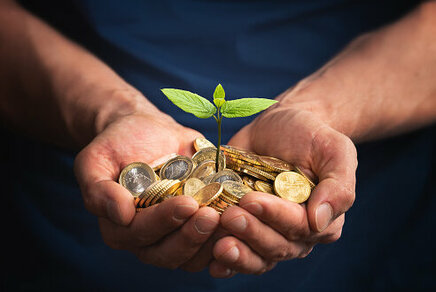
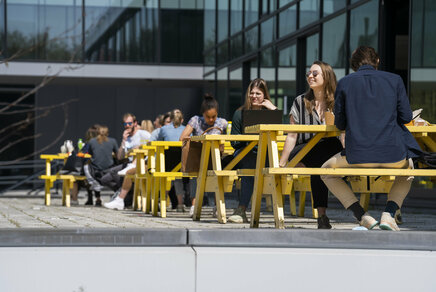
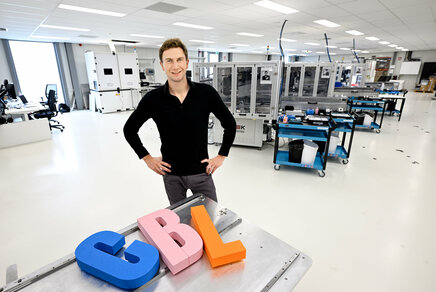