Digital twin development for improved operation of batch process systems
Carlos Mendez Blanco defended his PhD thesis at the department of Electrical Engineering on September 21st.
![[Translate to English:] [Translate to English:]](https://assets.w3.tue.nl/w/fileadmin/_processed_/a/8/csm_Blanco%20Banner%20image_15bed454f7.jpg)
The emergence of digital twins has revolutionized various industries, offering unprecedented insights into complex systems and processes. A digital twin is a virtual representation of a physical object, system, or process that allows real-time monitoring, analysis, and optimization. In recent years, digital twins have become instrumental in enhancing efficiency, reducing costs, and mitigating risks across a wide range of industries. One crucial aspect of digital twins is model calibration, where parameter estimation plays a pivotal role in ensuring accurate and reliable simulations. For his PhD research, Carlos Mendez Blanco developed a way to improve the estimation of key parameters that affect the accuracy of digital twin models.
Digital twins are used in industries like manufacturing, healthcare, energy, transportation, and more. By creating a virtual replica of a physical entity, companies gain a deeper understanding of its behavior, enabling them to make data-driven decisions and improve overall performance.
In manufacturing, digital twins facilitate predictive maintenance, optimizing production processes, and reducing downtime. In healthcare, they aid in patient-specific treatments, simulating the effects of various interventions on an individual's health. The energy sector leverages digital twins to optimize resource allocation and enhance energy efficiency, while transportation industries use them to improve logistics and traffic management.
Real-world scenarios
Key to the effectiveness of digital twins is their ability to accurately simulate real-world scenarios. Model calibration, a critical step in creating digital twins, involves adjusting model parameters to match real-world observations.
Parameter estimation is a core aspect of model calibration that plays a central role in ensuring the digital twin behaves like its physical counterpart. The process involves comparing simulation results with real-time data, iterating on model parameters until a close match is achieved.
Effective parameter estimation requires the integration of advanced algorithms and optimization techniques. These methods analyze vast amounts of data, refine the models, and minimize the discrepancies between the digital twin's behavior and the physical system's performance. By accurately estimating parameters, companies can confidently rely on digital twins for making informed decisions, enhancing productivity, and reducing operational risks.
Benefits and challenges
The benefits of employing parameter estimation in model calibration are far-reaching. It leads to improved predictive capabilities, enabling companies to anticipate system behavior and potential failures.
This, in turn, allows for proactive maintenance and helps prevent costly downtime. Moreover, accurate calibration enhances system understanding, aiding in the discovery of previously unidentified inefficiencies or bottlenecks.
However, challenges persist in implementing parameter estimation effectively. The availability of high-quality data, the complexity of systems, and computational requirements pose significant hurdles. Therefore, in his research, Carlos Mendez Blanco develops a technique to improve parameter estimation in complex batch systems exploiting the stepwise nature of batch systems, focusing on a step at a time instead of the whole process to achieve better accuracy in the calibrated model.
Guidelines
Mendez Blanco proposes methodologies to verify whether various parameters in the digital twin can be estimated, as well as guidelines to ensure that the data extracted from the system still provides high quality information.
These novel developments are tested in the calibration and optimization of a real-world digital twin of an industrial process for the manufacture of resins.
The advent of digital twins has revolutionized industry by offering real-time insights, enhancing decision-making processes, and optimizing performance across various sectors. Parameter estimation, as an integral part of model calibration, plays a vital role in ensuring the digital twin accurately replicates its physical counterpart.
By effectively estimating parameters, companies can harness the full potential of digital twins, improving predictive capabilities, optimizing processes, and bolstering safety in the era of Industry 4.0.
Title of PhD thesis: Digital twin development for improved operation of batch process systems. Supervisors: Paul Van den Hof and Leyla Özkan.
Media contact
Latest news
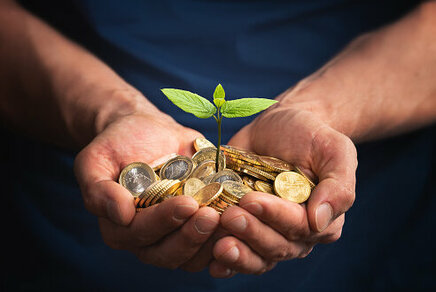
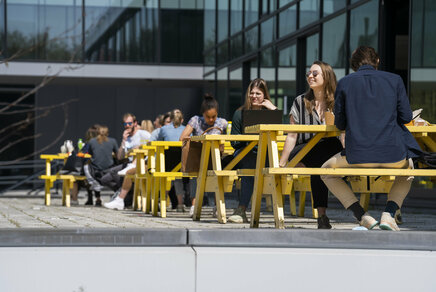
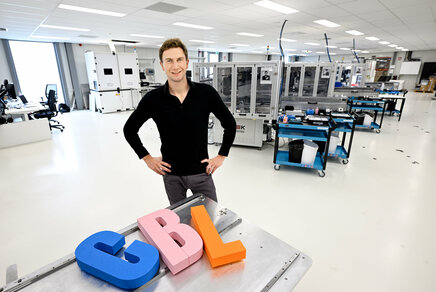