Improving electricity trading algorithms to minimize risk and cost
PhD researcher Sumeyra Demir has improved the forecasting accuracies of electricity prices and developed trading algorithms for the short-term electricity markets.
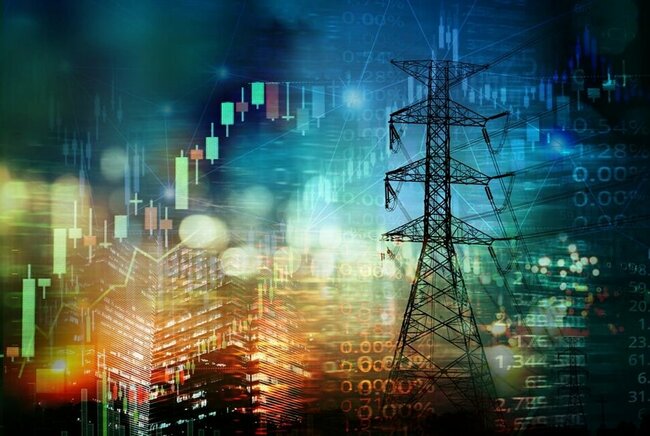
There is an urgent need to move away from fossil fuels, tackle climate change, and achieve energy independence nowadays. Though necessary, the transition away from polluting, yet stable energy sources raises technological and economic challenges. For her PhD research, Sumeyra Demir has offered novel AI-based solutions to tackle some of these challenges. Her solutions help ensure the stability of electrical grids and the efficiency of short-term electricity markets. Her research is funded by an innovative energy company, Scholt Energy. She defended her thesis at the department of Electrical Engineering on June 7th.
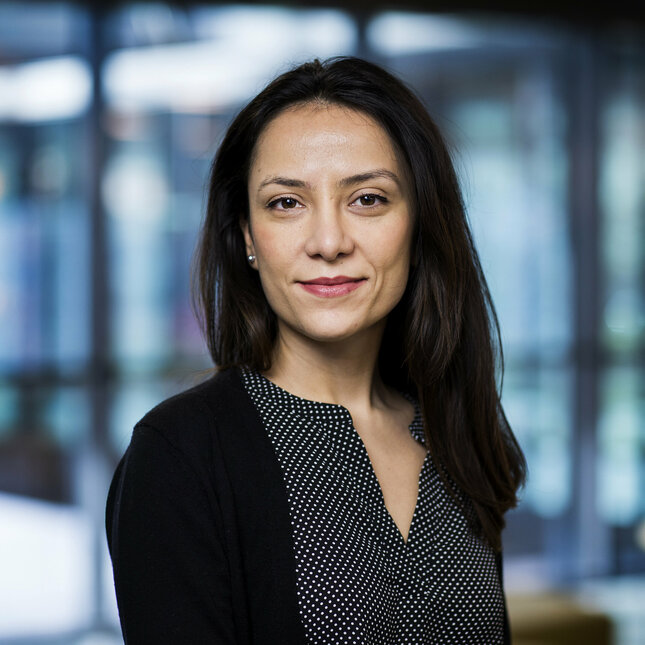
The supply from renewable energy sources is dependent on weather conditions which are difficult to forecast accurately. Given this limitation, electricity traders have been forced to adjust their trading strategies from long-term to short-term trading, to be able to use more recent and reliable forecasts. This shift in liquidity, however, has been insufficient to offset the additional risk created by renewable energy productions and combat increasing price deviations emerging across markets.
Arbitrage trading strategies offer the opportunity to reduce price differences between markets and increase market liquidity and efficiency while yielding profits for traders. Arbitrage trading strategies employ analysis to determine reasonable price levels at which to enter and exit trades without making long-term physical commitments.
Arbitrage trading electricity is more difficult than arbitrage trading other commodities. The uncertainty from renewable sources mentioned above impacts the accuracy of electricity price forecasts, increasing the risk for traders. Short trading windows, during which trades are executed, further complicate the optimisation of trading opportunities. Novel methods are thus needed to predict prices accurately and make trading decisions optimally.
Automated and Optimized Arbitrage Trading Agents
In her research, Sumeyra, firstly, has significantly increased the accuracy of short-term electricity market price forecasts by using novel methods, such as technical indicators and data augmentation. Technical indicators, such as moving averages and Bollinger bands, capture the behavioural biases of traders. Using these features allows machine learning models to better capture trading signals and thus better predict prices. Similarly, data augmentation methods employing autoencoders and generative adversarial networks are found to successfully expand data size. An increased data size reduces the error of models, reducing the impacts of data drift.
Secondly, Sumeyra has deployed novel algorithms to significantly increase trading agents’ profits. Trading agents are automated decision makers capable of placing profitable trades. Instead of training humans to recognise profitable entry and exit points, by feeding labelled data into a reinforcement learning algorithm a machine is taught to spot these patterns quickly, efficiently, and at scale. Sumeyra has developed novel functions to help the reinforcement algorithm extract valuable information from noisy trading data.
Energy Transition
In implementing arbitrage trading strategies, robust trading agents can help in the transition away from fossil fuels, combating climate change and helping attain energy independence. Strategies for arbitrage trading provide the chance to boost market liquidity, improve market efficiency, eliminate price discrepancies across markets, and make a profit. Three of these opportunities counteract the challenges renewable energy creation creates. Helping arbitragers, thus, presents an opportunity to accelerate the energy transition.
Title of PhD-thesis: Statistical Arbitrage Trading on Electricity Markets Using Deep Reinforcement Learning. Supervisors: Koen Kok (TU/e), and dr. Nikolaos Paterakis (TU/e). Other main parties involved: Scholt Energy.