
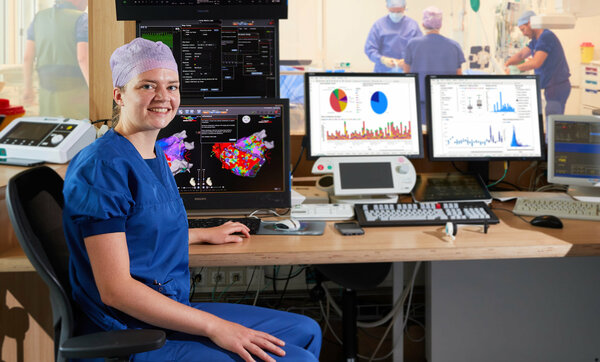
Marloes de Winter
Project: Revolutionizing Cardiovascular Research: a Dynamic Dashboard for Data Visualization and Analysis for the COMBAT-VT study
For post-myocardial infarction (MI) ventricular tachycardia (VT) patients, primary risk stratification is based on left ventricular ejection fraction (LVEF) and symptoms. This proves to be insufficient in identifying patients at high risk. LVEF is a general measurement which barely encompasses the VT development complexity. This complexity might be better interpreted by visualizing and analysing the different routinely obtained data of MI patients. Through the strong collaboration within e/MTIC in this EngD QME project, we create an integration of technical and clinical practice. In hospitals an abundance of data is created but not always used to its full potential. Coupling different multimodal data and analysing their time-evolving trends in a dashboard can unravel new useful insights. In addition, the implementation of this data management workflow and dashboard necessitates active engagement with a multi-disciplinary team. Collaborating with PhDs allows us to enhance the depth and quality of our analyses with patient-specific models, based on obtained data. Furthermore, actively involving stakeholders such as clinicians and ICT specialists enables an understanding of the practical implications, feasibility and real-world applicability of our findings.
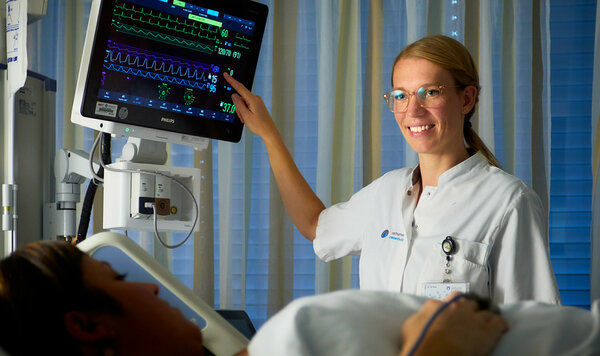
Tineke de Vries
Project: Design, implementation and application of a perioperative high-resolution monitoring database
Vital sings of patients are continuously monitored during surgery and on the Intensive Care. Availability of this data in high resolution gains interest for better clinical monitoring, continuous quality surveillance, and for research such as training prediction models with artificial intelligence. The aim of my project is to design and implement a high-resolution database of all vital signs that are available on the patient bedside monitor, such as heart activity, blood pressure, and respiration. This data is automatically saved and stored, and data from different medical devices (e.g. the patient monitor and mechanical ventilator) are synchronized. The database will be connected to the electronic health record in a data lake, to provide context to the data. Three pilots are setup to further develop the workflow for using the data within clinical and research settings. For this, we collaborate with the AI expertise centre of the Catharina Hospital. As EngD Qualified Medical Engineer trainee based in the Catharina Hospital and TU/e, I closely collaborate with PhD students with Medicine and Engineering backgrounds as well as hospital specialists and industry partner Philips. Within these collaborations we combine different areas of expertise which allows us to design an optimal solution for all partners.
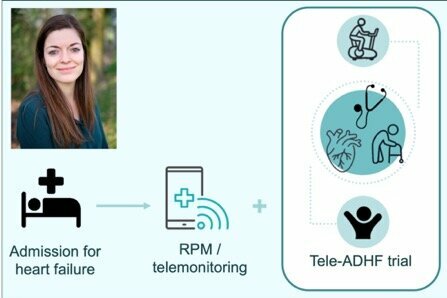
Mayke van Leunen
Project: PATIENCE - Telerehabilitation in patients after hospitalisation for Acute Decompensated Heart Failure (Tele-ADHF)
Heart failure (HF) is a clinical syndrome characterized by significant morbidity and mortality, poor quality of life, high costs, and frequent hospitalisations. More than 64 million people worldwide are living with HF and this prevalence is expected to grow due to the global ageing. To improve quality of life and physical function, and to prevent of (re)hospitalisation in those suffering from heart failure, cardiac rehabilitation (CR) in needed. Currently under 5% of HF patients receive CR in the Netherlands due to non-referral, anxiety or depression symptoms in patients or physically not being able to reach the rehabilitation centre. In our study, the Tele-ADHF trial, we expect to reach more HF patients with a home-based rehabilitation programme involving a physical and occupational therapist, dietician and psychologist. Multiple technical innovations are used to support the program: a digital platform for telemonitoring, video training and video coaching; a chatbot for monitoring the food intake; and the Philips activity watch to give tailored advice on daily physical exercises. This research is made possible by the collaboration of the Cardiology department of the Máxima Medical Centre (MMC), Industrial Design department of the TU/e and Philips Research.
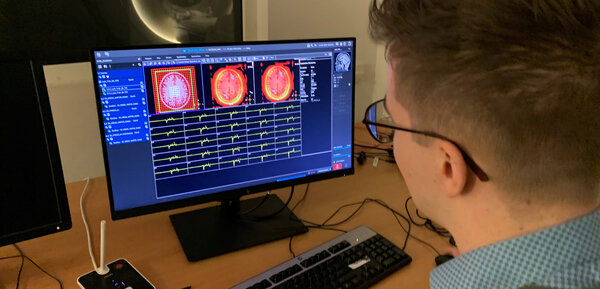
Dennis van de Sande
Project: Spectralligence
Spectroscopy is a crucial technology in fields like chemistry, medicine, and environmental and safety services. It uses the electromagnetic spectrum to generate unique fingerprints of molecular structures. But, the process requires human experts to acquire, process, and analyse spectral data, which makes the overall workflow very complex for non-experts and relatively time-consuming. Spectralligence is changing that by introducing artificial intelligence (AI) into the spectroscopy process. Our team at TU/e is working with Philips, Maastricht UMC, and other spectroscopy companies to develop cross-domain validated neural networks that aims to revolutionize spectroscopy across industries. Our TU/e team focuses mainly on Magnetic Resonance Spectroscopy (MRS), a non-invasive technique used to study the metabolism of the human brain. With partners from different spectroscopic domains, we are able to bring fresh perspectives and innovative ideas to the table. Some of the AI solutions we're currently developing include generative models for spectral data generation, models for artifact removal, and classification tools for clustering or determining the quality of spectra. Visit our website to stay up-to-date with our latest achievements and join us on our journey to revolutionize spectroscopy with Spectralligence!

Jiali Xie
Project: Multimodal unobtrusive monitoring of snoring and sleep apnea detection including acoustic signal analysis
Obstructive sleep apnea (OSA) is one of the most common sleep disorders, which affects 9% to 17% of the general adult population by estimation. Polysomnography (PSG) is the gold standard for diagnosing OSA. However, PSG requires costly sleep center facilities, overnight hospitalization, and instrument disrupting sleepers. Thus, there is an urge to have unobtrusive and reliable OSA screening techniques. This project is to investigate and develop an OSA monitoring platform with multimodal unobtrusive measurements that can be used in a home environment. In this project, multiple signals were collected from Kempenhaeghe sleep center. A PhD candidate and researchers from Kempenhaeghe, TU/e, and Phillips cooperate to study and develop OSA screening methods based on those signals. Our first focus is on audio signal, we detected and used the snoring sound from audio to estimate OSA severity. Then, we used algorithms based on ECG signal to detect sleep disorder breathing. Next, the focus will be accurate OSA detection combining multimodal signals including acoustic signal. Multiple signal processing and machine learning technologies are applied in this project.