Jim Portegies
Department / Institute
Group
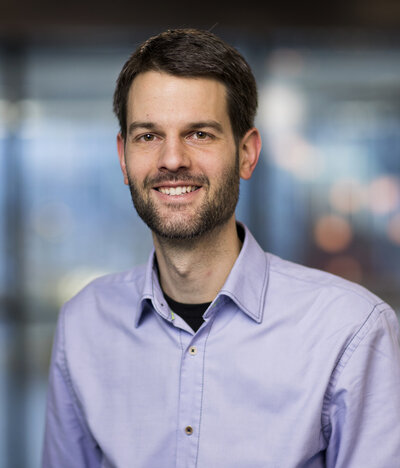
RESEARCH PROFILE
Jim Portegies is an Assistant Professor in the Applied Analysis group of the Centre for Analysis, Scientific computing and Applications (CASA) at Eindhoven University of Technology (TU/e).
Jim Portegies works in the mathematical fields of analysis, measure theory and geometry, and applies techniques from these fields to problems in machine-learning and artificial intelligence. He has applied techniques from spectral geometry to prove guarantees on performance of nonlinear dimensionality reduction algorithms. Currently, he is investigating how to design algorithms that mimic how humans and animals learn. Despite the large number of recent advances in artificial intelligence, humans still outperform machines in many tasks. The central question of how to design machines that learn like humans is still wide open. The answer may lie in universal learning algorithms. Such algorithms are simple, efficient and can be applied to a broad variety of signals and tasks and are conjectured to exist in the depths of the human brain.
Conjecturally, in the depths of the human brain runs an immensely powerful, simple, efficient and task- and signal-independent learning algorithm. It is my ultimate aim to use mathematics to uncover and develop such algorithms.”
ACADEMIC BACKGROUND
Jim Portegies obtained his MSc in Industrial and Applied Mathematics and Applied Physics from the TU/e in 2009. He spent the 2007-2008 academic year as an exchange student at the University of Bonn, Germany. He received his PhD in Mathematics from the Courant Institute of Mathematical Sciences in New York. In the Fall of 2013, he spent a semester at NYU Shanghai, in Shanghai, China. After completing his PhD in 2014, he spent two years a postdoc at the Max Planck Institute for Mathematics in the Sciences in Leipzig, Germany until he returned to the TU/e as an assistant professor in Mathematics in 2016. Jim is a member of the TU/e Young Academy of Engineering.
Recent Publications
-
Feedforward Tuning with Input Nonlinearities
(2023) -
PDE-Based Group Equivariant Convolutional Neural Networks
Journal of Mathematical Imaging and Vision (2023) -
Waterproof: educational software for learning how to write mathematical proofs
arXiv (2022) -
Data-driven compensation of unmodeled dynamics for complex mechatronic systems
(2022) -
Feedforward control in the presence of input nonlinearities
IFAC-PapersOnLine (2022)
Current Educational Activities
Ancillary Activities
No ancillary activities