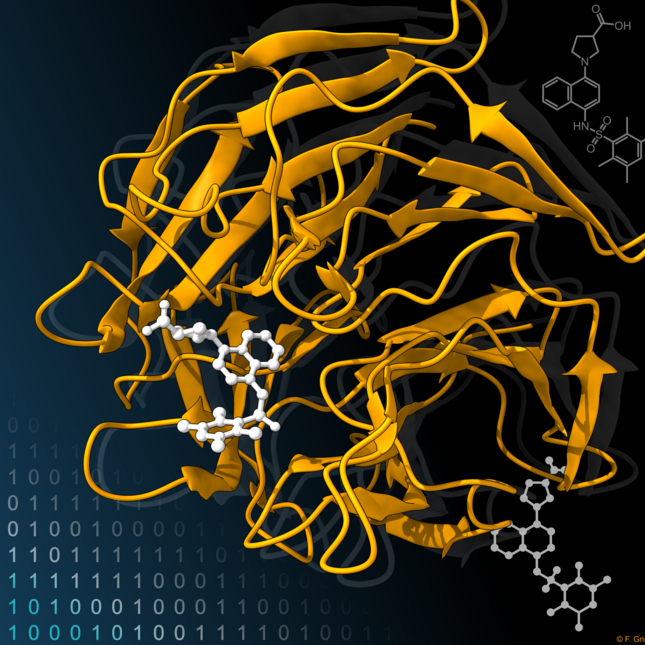
Discovering innovative molecules with the desired bioactivity is an essential step to develop new drugs and gather a greater understanding of biological systems. Machine learning bears promise to accelerate the molecule discovery pipeline, by allowing for a time- and cost-efficient navigation of the incredibly vast chemical universe. The Molecular Machine Learning team at TU/e, led by Dr. Francesca Grisoni, develops and applies data-driven methods to design novel molecular entities and unveil structure-activity relationships of small molecules and peptides. With research located at the interface between chemical biology and AI, our final mission is to develop cutting-edge computational tools to augment human intelligence in molecular discovery and drug development.
Our current areas of research are:
- Generative deep learning, to design novel molecules with desired properties from scratch without the need for human-engineered construction rules.
- AI-driven multi-objective compound discovery, e.g., for selectivity optimization and polypharmacology.
- Development and analysis of molecular representations suited to machine learning in chemical biology.
- Low-data regime machine learning, to allow molecule discovery on unexplored macromolecular targets.