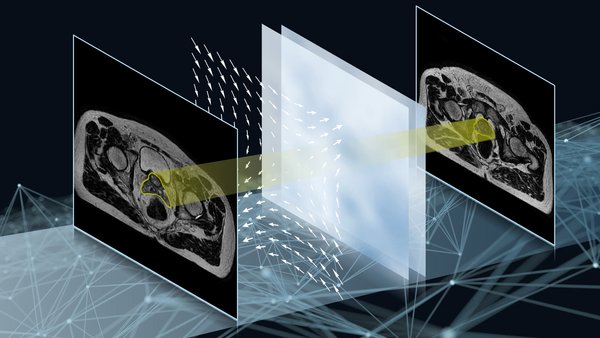
This research line focuses on developing and evaluating novel deep learning approaches to reconstruct and process medical images, thereby enabling the use of personalized, image-guided treatments on a larger scale. Deep learning has already shown great potential to improve the reconstruction of images from raw measurements, automatically delineate anatomical structures, and enable fast registration of medical images. Before such deep learning approaches can be adopted in clinical settings, various questions still need to be answered. How can we collect sufficient amounts of labelled imaging data to train deep learning methods? And how can we ensure the robustness of these methods across different imaging modalities, scanner settings and patient populations?
Current research topics include:
- Low-dose (cone-beam) CT imaging and deep learning-based image reconstruction
- Deep learning for deformable image registration
- Deep learning for image segmentation
- Applications in surgical navigation and robotics, virtual treatment planning, 3D printing, and adaptive radiotherapy