Mike Holenderski
Department / Institute
Group
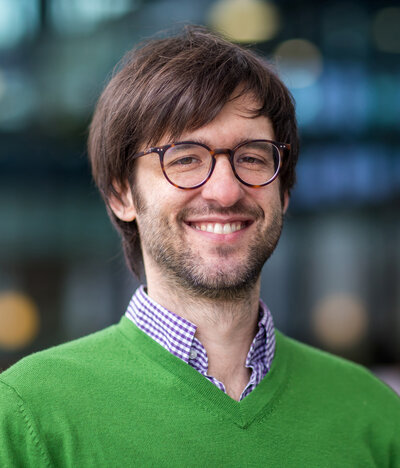
RESEARCH PROFILE
Mike Holenderski is an Assistant Professor at the Eindhoven University of Technology at the System Architecture and Networking group. His research focus is on, machine learning which has been shown to perform well in various domains, some- times even outperforming humans on tasks such as image classification. Usually, the data that the machine learning systems are trained and evaluated on are static and relatively clean. In reality, however, the data is often noisy, corrupted, partially missing and coming from dynamic environments which are changing over time.
Reliable machine learning aims at developing models which are robust to noise and missing data, and can detect and adapt to changes in the processes that they monitor and control. The relevant questions include: How can a system measure its own performance and detect when its predictions are not accurate, e.g. because the behavior of the monitored process has changed or because the input is very different from previous observations? How can it provide safe predictions when it cannot be certain about the accuracy? How can a system adapt to the changes in the environment or when being deployed in a new context? How can a machine learning agent deal with corrupt or missing data?
I am interested in the design and applications of reliable machine learning in industrial systems for optimizing the manufacturing and maintenance processes.”
ACADEMIC BACKGROUND
Mike Holenderski received his PhD in 2012 on the topic of real-time systems. Since then he has been working on Machine Learning. His current interest is Reliable Machine Learning, where the goal is to perform machine learning tasks, such as failure prediction or wear estimation, using real industrial data which is often noisy, corrupted, partially missing or coming from dynamic environments which change over time. He is interested in putting the theory in practice, which he has been doing in collaborations with industry through various European and national projects.
Recent Publications
-
Out-of-Distribution Generalisation with Symmetry-Based Disentangled Representations
(2023) -
Equivariant Representation Learning in the Presence of Stabilizers
(2023) -
Equivariant Representations for Non-Free Group Actions
(2022) -
Quantifying and Learning Linear Symmetry-Based Disentanglement
(2022) -
Predictive discarding of wafers based on power leakage predictions from single layer misalignment data
Procedia Computer Science (2022)
Current Educational Activities
Ancillary Activities
No ancillary activities